Uso de redes neuronales artificiales y señales electromiográficas para el diseño y control de prótesis inteligentes
DOI:
https://doi.org/10.56162/transdigital346Palabras clave:
señales electrmiográficas, redes neuronales artificiales, prótesis, inteligencia artificialResumen
Cada año, muchas personas alrededor del mundo pierden extremidades corporales debido a enfermedades, accidentes u otras circunstancias. Hoy en día, la tecnología permite desarrollar prótesis de poco coste económico y alta eficiencia en su respuesta. Estas nuevas tecnológicas ayudan al momento de diseñar prótesis, pues no se enfocan únicamente en la parte estética, sino en que sean funcionales y emulen los movimientos naturales del miembro perdido. En este artículo se presenta una revisión de las técnicas más utilizadas para generar prótesis, además de centrarse en aquellas que utilizan esquemas de inteligencia artificial, como las redes neuronales y elementos biométricos para su control.
Citas
Abu, M. A., Rosleesham, S., Suboh, M. Z., Yid, M. S. M., Kornain, Z., & Jamaluddin, N. F. (2020). Classification of EMG signal for multiple hand gestures based on neural network. Indonesian Journal of Electrical Engineering and Computer Science, 17(1), 256-263. http://doi.org/10.11591/ijeecs.v17.i1.pp256-263
Ahsan, M. R., Ibrahimy, M. I., & Khalifa, O. O. (2011). Electromygraphy (EMG) signal-based hand gesture recognition using artificial neural network (ANN) [Sesión de congreso]. 4th international conference on mechatronics (ICOM), Kuala Lumpur, Malaysia. https://ieeexplore.ieee.org/document/5937135
Akmal, M., Qureshi, M. F., Amin, F., Rehman, M. Z. U., & Niazi, I. K. (2021). SVM-based Real-Time Classification of Prosthetic Fingers using Myo Armband-acquired Electromyography Data [Sesión de congreso]. 21st International Conference on Bioinformatics and Bioengineering (BIBE), Kragujevac, Serbia. . https://ieeexplore.ieee.org/document/9635461
Aswath, S., Tilak, C. K., Suresh, A., & Udupa, G. (2014). Human gesture recognition for real-time control of humanoid robot. International Journal of Advances in Mechanical & Automobile Engineering, 1(1), 96-100. http://iieng.org/images/proceedings_pdf/6717E0314213.pdf
Avilés-Mendoza, K., Gaibor-León, N. G., Asanza, V., Lorente-Leyva, L. L., & Peluffo-Ordóñez, D. H. (2023). A 3D Printed, Bionic Hand Powered by EMG Signals and Controlled by an Online Neural Network. Biomimetics, 8(2), 255. https://doi.org/10.3390/biomimetics8020255
Aviles, M., Sánchez-Reyes, L. M., Fuentes-Aguilar, R. Q., Toledo-Pérez, D. C., & Rodríguez-Reséndiz, J. (2022). A novel methodology for classifying EMG movements based on SVM and genetic algorithms. Micromachines, 13(12), 2108. https://doi.org/10.3390/mi13122108
Bak?rc?o?lu, K., & Özkurt, N. (2020). Classification of Emg Signals Using Convolution Neural Network. International Journal of Applied Mathematics Electronics and Computers, 8(4), 115-119. https://doi.org/10.18100/ijamec.795227
Calderón-Córdova, C., Ramírez, C., Barros, V., Quezada-Sarmiento, P. A., & Barba-Guamán, L. (2016). EMG signal patterns recognition based on feedforward Artificial Neural Network applied to robotic prosthesis myoelectric control [Sesión de congreso]. Future Technologies Conference (FTC), San Francisco, United States of America.
Chowdhury, M. Z. A., & Roy, D. P. (2020). Circuit Design and Analysis of an Electromyography (EMG) Signal Acquisition System [Sesión de congreso]. 2nd International Conference on Electrical, Control and Instrumentation Engineering (ICECIE), Kuala Lumpur, Malaysia.
Cimolato, A., Driessen, J. J., Mattos, L. S., De Momi, E., Laffranchi, M., & De Michieli, L. (2022). EMG-driven control in lower limb prostheses: A topic-based systematic review. Journal of NeuroEngineering and Rehabilitation, 19(1), 43. https://doi.org/10.1186/s12984-022-01019-1
Ghaffar Nia, N., Kaplanoglu, E., & Nasab, A. (2023). Evaluation of artificial intelligence techniques in disease diagnosis and prediction. Discover Artificial Intelligence, 3(1), 5.
Gómez-Correa, M., & Cruz-Ortiz, D. (2022). Low-cost wearable band sensors of surface electromyography for detecting hand movements. Sensors, 22(16), 5931. https://doi.org/10.3390/s22165931
Ibrahimy, M. I., Ahsan, R., & Khalifa, O. O. (2013). Design and optimization of Levenberg-Marquardt based neural network classifier for EMG signals to identify hand motions. Measurement Science Review, 13(3), 142-151. https://doi.org/10.2478/msr-2013-0023
Jia, R., Yang, L., Li, Y., & Xin, Z. (2021). Gestures recognition of sEMG signal based on Random Forest [Conferencia]. IEEE 16th Conference on Industrial Electronics and Applications, Chengdu, China.
Jorgensen, C., Wheeler, K., Stepniewski, S., & Norvig, P. (2000). Bioelectric control of a 757 class high fidelity aircraft simulation. Página web oficial de NTRS-NASA Technical Reports Server. https://ntrs.nasa.gov/citations/20010083844
Kiguchi, K., & Hayashi, Y. (2012). An EMG-based control for an upper-limb power-assist exoskeleton robot. IEEE Transactions on Systems, Man, and Cybernetics, Part B (Cybernetics), 42(4), 1064-1071.
Naim, A. M., Wickramasinghe, K., De Silva, A., Perera, M. V., Lalitharatne, T. D., & Kappel, S. L. (2020). Low-cost active dry-contact surface emg sensor for bionic arms [Sesión de congreso]. 2020 IEEE International Conference on Systems, Man, and Cybernetics, Toronto, Canada.
McDonald, C. L., Westcott-McCoy, S., Weaver, M. R., Haagsma, J., & Kartin, D. (2021). Global prevalence of traumatic non-fatal limb amputation. Prosthetics Orthot Int, 45(2),105-114.
Mohshim, S. A., Arshad, A. F., Fadzir, M. F., & Fadzly, M. K. (2020). Development of prosthetic hand using Arduino for handicap person. AIP Proceedings, 2291(1) https://doi.org/10.1063/5.0023133
Nguyen, M., Gia, T. N., & Westerlund, T. (2021). EMG-based IoT system using hand gestures for remote control applications [Sesión de congreso]. 2021 IEEE 7th World Forum on Internet of Things (WF-IoT), New Orleans, United States of America.
Precup, R. E., Teban, T. A., Albu, A., Borlea, A. B., Zamfirache, I. A., & Petriu, E. M. (2020). Evolving fuzzy models for prosthetic hand myoelectric-based control. IEEE Transactions on Instrumentation and Measurement, 69(7), 4625-4636. https://ieeexplore.ieee.org/document/9047868
Raheema, M. N., Hussain, J., & Al-Khazzar, A. M. (2020). Design of an intelligent controller for myoelectric prostheses based on multilayer perceptron neural network. IOP Conference Series Materials Science and Engineering, 671(1), 012064.
Rahimian, E., Zabihi, S., Atashzar, S. F., Asif, A., & Mohammadi, A. (2020). Surface EMG-Based Hand Gesture Recognition via Hybrid and Dilated Deep Neural Network Architectures for Neurorobotic Prostheses. Journal of Medical Robotics Research, 5(01n02), 2041001. https://doi.org/10.1142/S2424905X20410019
Soares, A., Andrade, A., Lamounier, E., & Carrijo, R. (2003). The development of a virtual myoelectric prosthesis controlled by an EMG pattern recognition system based on neural networks. Journal of Intelligent Information Systems, 21, 127-141. https://link.springer.com/article/10.1023/A:1024758415877
Tinoco-Varela, D., Ferrer-Varela, J. A., Cruz-Morales, R. D., & Padilla-García, E. A. (2022). Design and Implementation of a Prosthesis System Controlled by Electromyographic Signals Means, Characterized with Artificial Neural Networks. Micromachines, 13(10), 1681. https://doi.org/10.3390/mi13101681
Toro-Ossaba, A., Jaramillo-Tigreros, J., Tejada, J. C., Peña, A., López-González, A., & Castanho, R. A. (2022). LSTM recurrent neural network for hand gesture recognition using EMG signals. Applied Sciences, 12(19), 9700. https://doi.org/10.3390/app12199700
Triwiyanto, T., Caesarendra, W., Purnomo, M. H., Su?owicz, M., Wisana, I. D. G. H., Titisari, D., Lamidi, L., & Rismayani, R. (2022). Embedded machine learning using a multi-thread algorithm on a Raspberry Pi platform to improve prosthetic hand performance. Micromachines, 13(2), 191. https://doi.org/10.3390/mi13020191
Unanyan, N. N., & Belov, A. A. (2021). Design of upper limb prosthesis using real-time motion detection method based on EMG signal processing. Biomedical Signal Processing and Control, 70, 103062. https://doi.org/10.1016/j.bspc.2021.103062
Vergaray, R. A., Del Aguila, R. F., Avellaneda, G. A., Palomares, R., Cornejo, J., & Cornejo-Aguilar, J. A. (2021). Mechatronic System Design and Development of iROD: EMG Controlled Bionic Prosthesis for Middle-Third Forearm Amputee [Sesión de congreso]. IEEE Fifth Ecuador Technical Chapters Meeting (ETCM), Cuenca, Ecuador.
Xu, Y., Barbosa, P. C., Da Cunha Neto, J. S., Zhang, L., Shanmuganathan, V., de Albuquerque, V. H. C., & Pasupathi, S. (2023). Development of intelligent and integrated technology for pattern recognition in EMG signals for robotic prosthesis command. Expert Systems, 40(5), e13109. https://doi.org/10.1111/exsy.13109
Zhang, X. (2022). Application of artificial intelligence recognition technology in digital image processing. Wireless Communications and Mobile Computing, 2022(3), 1-10. https://doi.org/10.1155/2022/7442639
Zhang, Z., Yang, K., Qian, J., & Zhang, L. (2019). Real-time surface EMG pattern recognition for hand gestures based on an artificial neural network. Sensors, 19(14), 3170. https://doi.org/10.3390/s19143170
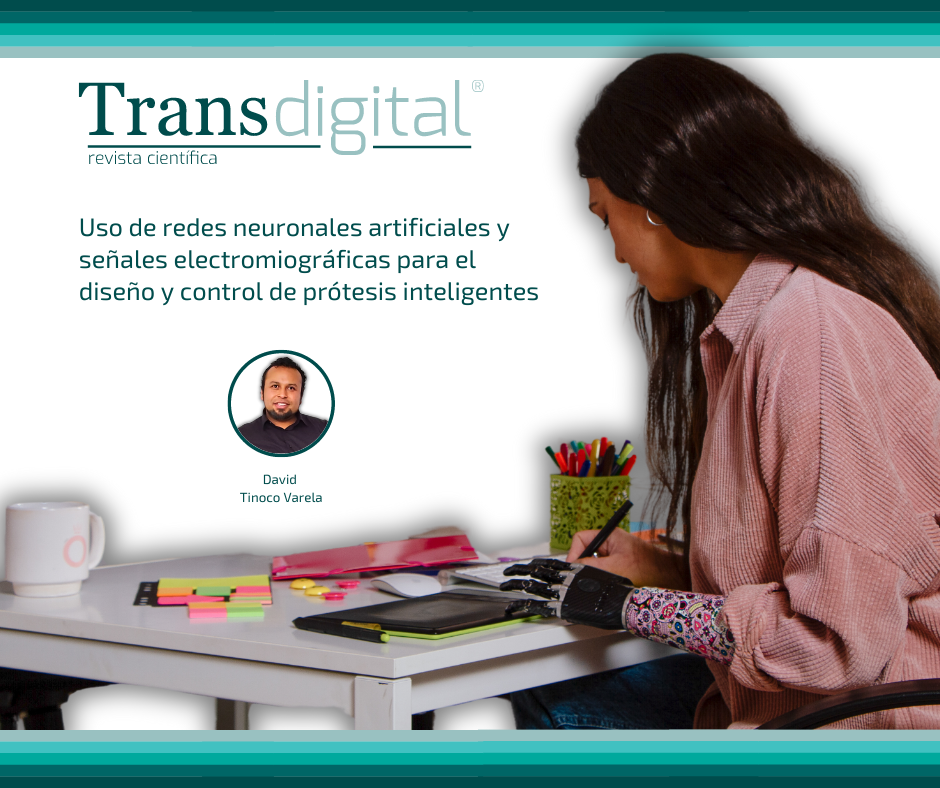
Autor de correspondencia
El autor de correspodencia se identifica con el siguiente símbolo: *Publicado
Cómo citar
Número
Sección
Categorías
Licencia
Derechos de autor 2024 David Tinoco Varela

Esta obra está bajo una licencia internacional Creative Commons Atribución 4.0.
Todos los artículos en Transdigital están licenciados bajo Creative Commons Attribution 4.0 International License Los autores poseen los derechos de autor y conservan los derechos de publicación sin restricciones.